Developing a Deep Learning Model to Automatically Detect Microscale Objects in Images and Videos
Project Overview
Our project will use an object detection algorithm designed for small objects to determine how many cysts are on the roots of soybean plants. We will also create a device to integrate image capturing with the machine learning algorithm. This will increase productivity on farms and production of soybeans by about 15-30%.
Our device will go to farmers to limit the number of unnecessary pesticides used on their plants. It will also go to geneticists and researchers to run tests and find if certain species of soybeans are resistant to the soybean cyst nematodes.
Team Members
Ethan Baranowski
Electrical Engineer, COM S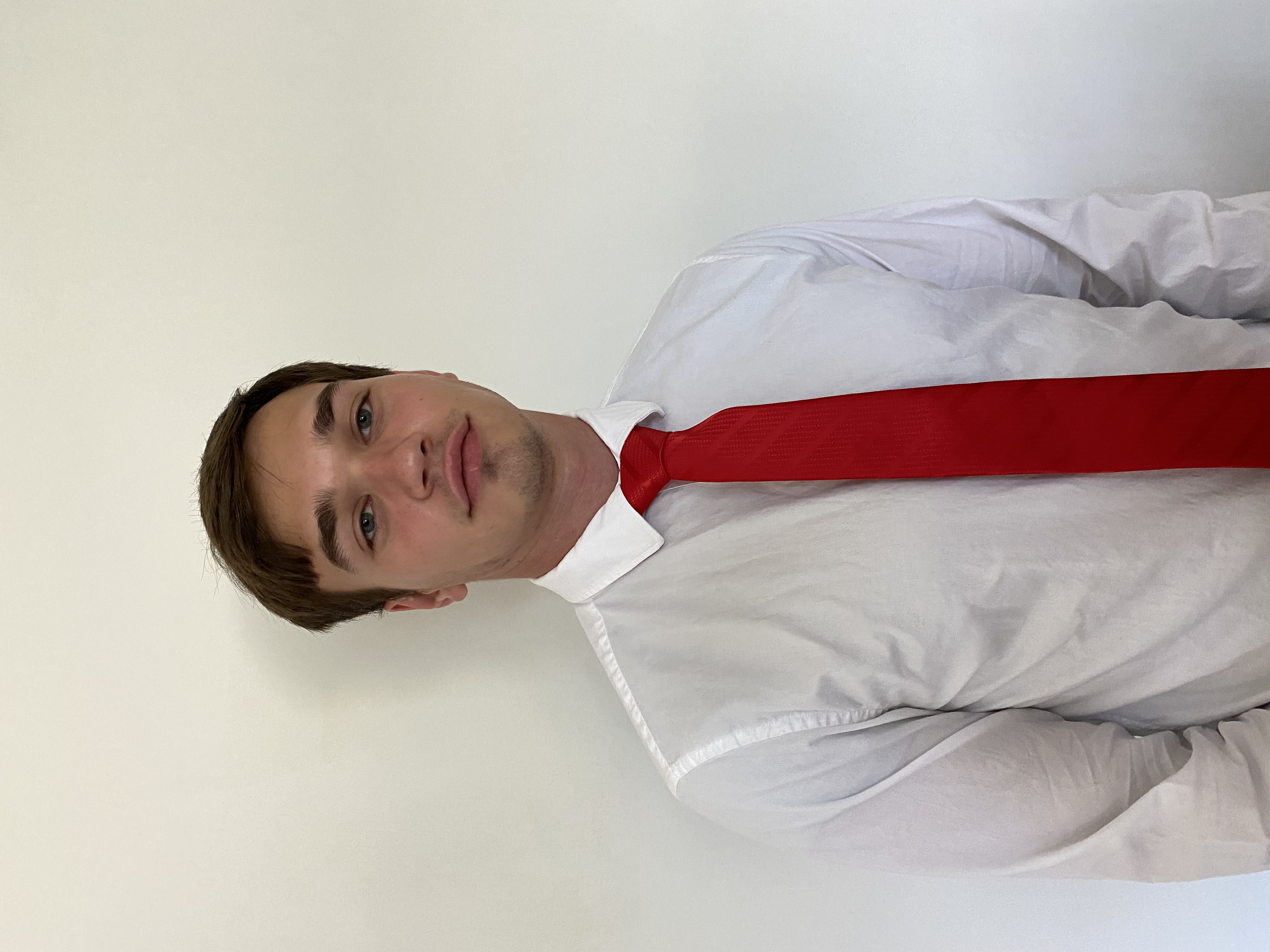
Ethan is an electrical engineer specializing in computer engineering and artificial intelligence. He hopes to be leader of an impactful team of engineers.
A fun fact is he is a member of the Theta Delta Chi fraternity.
Chris Cannon
Software Engineer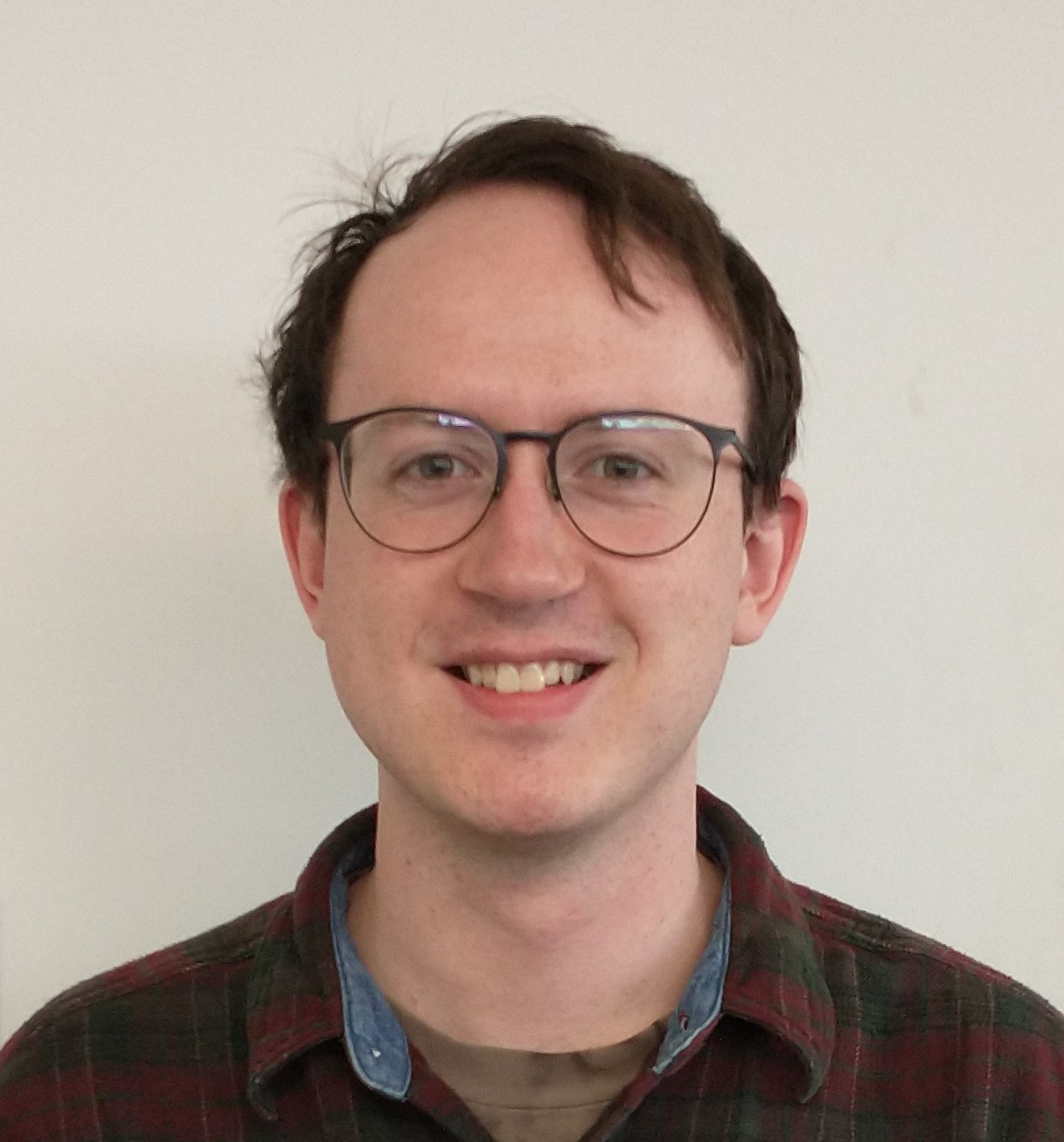
Chris is a senior in software engineering, interested in computer vision and application development.
In his free time, he enjoys playing trombone, reading, and playing video games.
Matthew Kim
Computer Engineer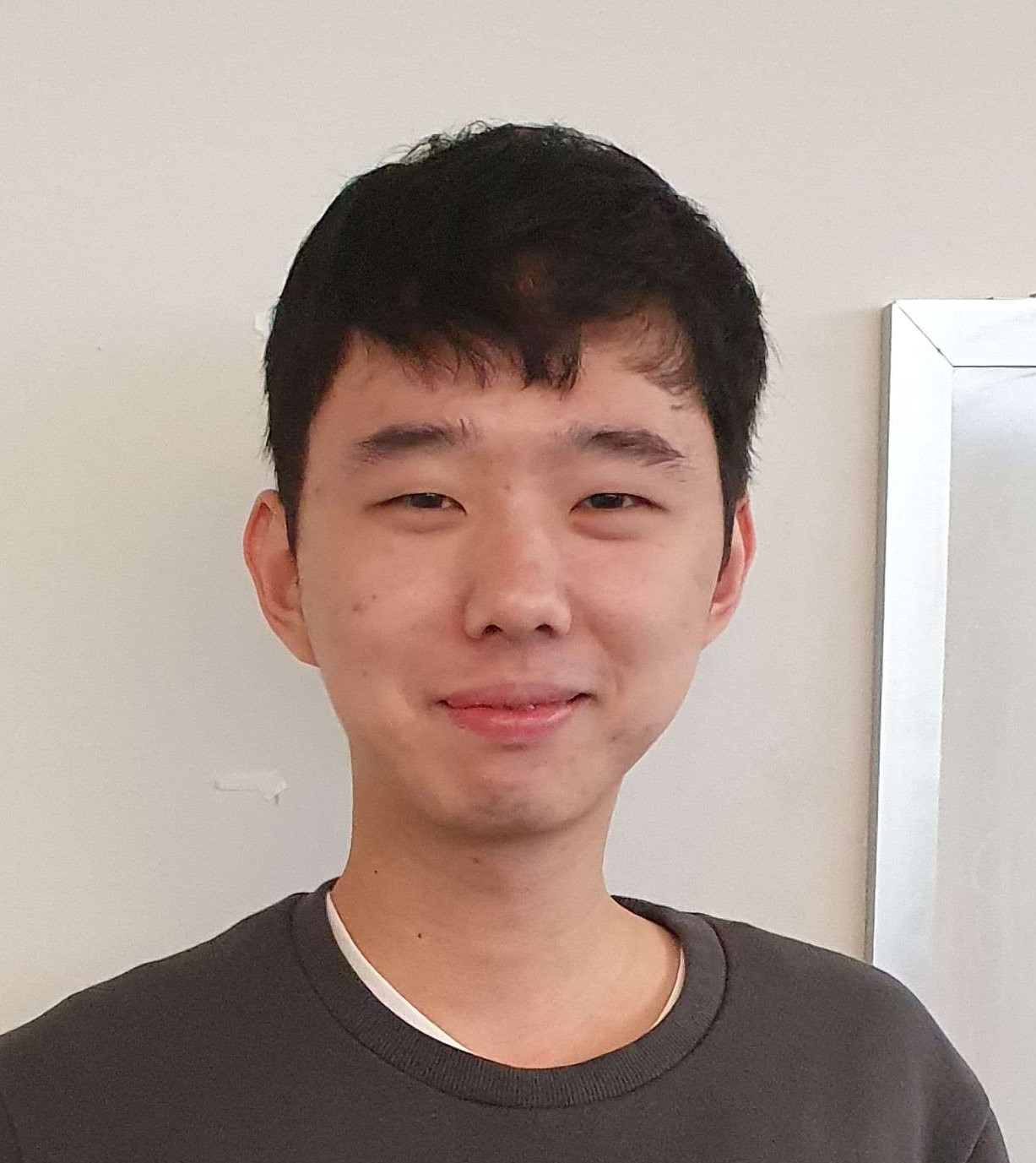
Matthew is a senior in Computer Engineering major. Currently, he is interested in Deep Learning and wants to learn more about the applications of the AI.
He knows how to play tennis and oboe.
Katie Moretina
Electrical Engineer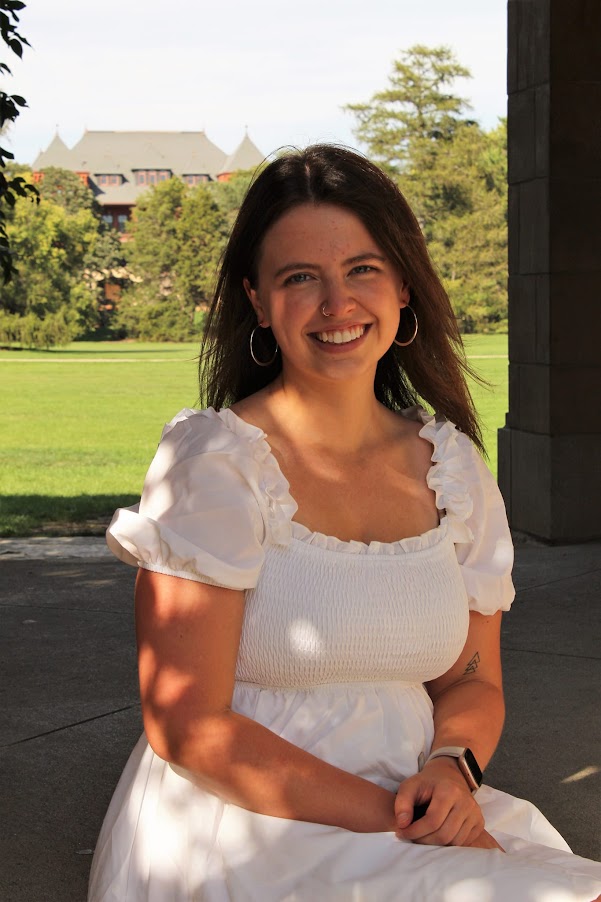
Katie is a senior in Electrical Engineering with an interest in control system design.
In her free time, she enjoys cooking, reading, and watching movies.
Design Documents
Design (491) DocumentDesign (491) Final Presentation
Project (492) Final Report
Project (492) Final Presentation
Lightning Talks
Design Lightning TalkProject Plan Lightning Talk
Requirements, Constraints, and Engineering Standards Lightning Talk
Testing Lightning Talk
Weekly Reports (491)
Report 1Report 2
Report 3
Report 4
Report 5
Report 6
Report 7
Report 8
Report 9
Biweekly Reports (492)
Biweekly Report 1 (Fall)Biweekly Report 2 (Fall)
Biweekly Report 3 (Fall)
Biweekly Report 4 (Fall)
Biweekly Report 5 (Fall)
Biweekly Report 6 (Fall)